Any test carried out on a particular population, must be able to calculate sensitivity, specificity, positive predictive value, and negative predictive value, to determine the usefulness of testing in detecting a particular disease or population characteristic. If we want to use a test to test certain characteristics in a sample population, what we need to know is:
- How likely is this test to detect existence certain characteristics of a person with such characteristics (sensitivity)?
- How likely is this test to detect absence certain characteristics of a person who do not have these characteristics (specificity)?
- How likely is it that someone who has the same test results positive truly have these characteristics (positive predictive value)?
-
How likely is it that a person whose test results negative truly do not have these characteristics (negative predictive value)?
These values are very important to calculate for determine whether a test is useful for measuring certain characteristics in a given population.
This article will show you how to calculate these values.
Step
Method 1 of 1: Counting Yourself
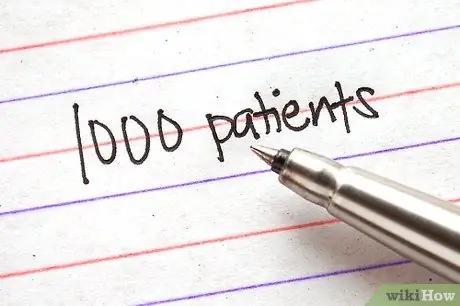
Step 1. Define the population to be sampled, for example 1000 patients in a clinic
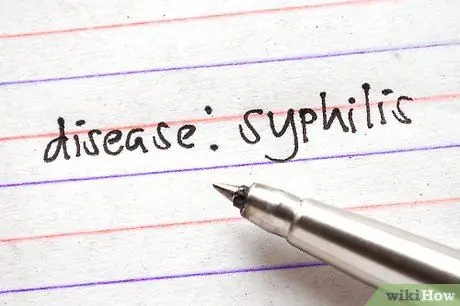
Step 2. Determine the desired disease or characteristic, eg syphilis
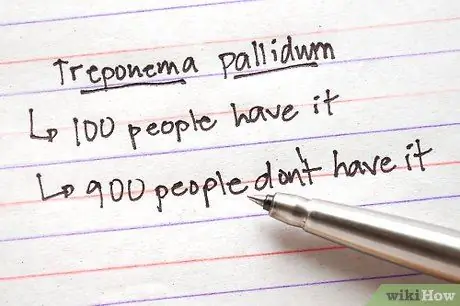
Step 3. Have a standard gold standard for determining disease prevalence or desired characteristics, eg dark-field microscopic documentation of the bacterium Treponema pallidum from syphilitic ulcer fragments, in collaboration with clinical findings
Use the gold standard test to determine who has the characteristics and who doesn't. As an illustration, let's say 100 people have the characteristic and 900 don't.
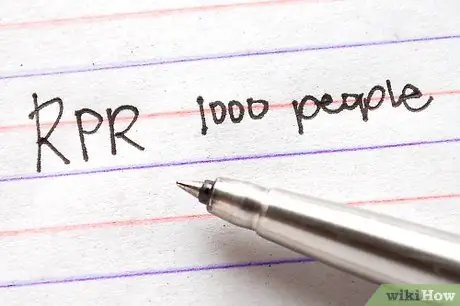
Step 4. Perform the test you are interested in to determine its sensitivity, specificity, positive predictive value, and negative predictive value for this population
Next, do the test for everyone in the sample population. For example, let's say this is a rapid plasma reagin test (RPR) to screen for syphilis. Use it to test 1000 people in a sample.
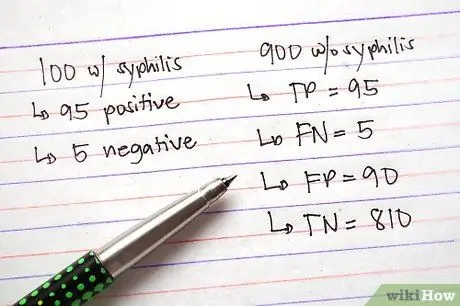
Step 5. For people who have the characteristics (as determined by the gold standard), record the number of people who tested positive and the number of people who tested negative
Do the same for people who do not have the characteristics (as defined by the gold standard). You will have four numbers. People who have the characteristics AND test results are positive are true positives (true positives or TP). People who have the characteristics AND test results are negative are false negatives (false negatives or FN). People who do not have the characteristics AND test results are positive are false positives (false positives or FP). People who do not have the characteristics AND test results are negative are true negatives (true negatives or TN). For example, suppose you have performed an RPR test on 1000 patients. Among the 100 patients with syphilis, 95 of them tested positive, while the remaining 5 were negative. Among the 900 patients who did not have syphilis, 90 tested positive, and the remaining 810 were negative. In this case, TP=95, FN=5, FP=90, and TN=810.
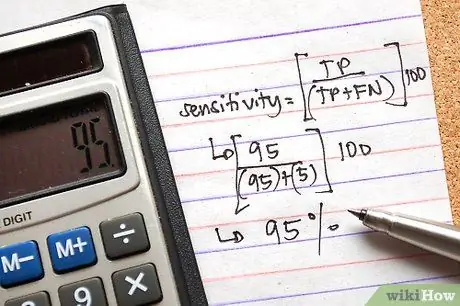
Step 6. To calculate sensitivity, divide TP by (TP+FN)
In the example above, the calculation is 95/(95+5)= 95%. Sensitivity tells us how likely the test is to give a positive result for a person who has the characteristic. Among all people who have the characteristic, what proportion test positive? The sensitivity of 95% is good enough.
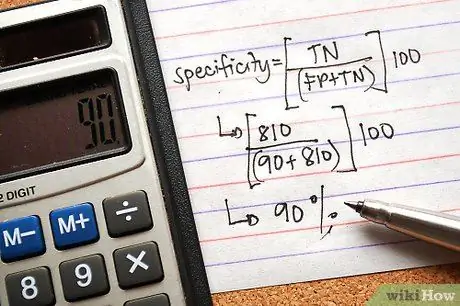
Step 7. To calculate specificity, divide TN by (FP+TN)
In the example above, the calculation is 810/(90+810)= 90%. Specificity tells us about the likelihood of a test giving a negative result in someone who does not have the characteristic. Among all people who do not have the characteristic, what proportion test negative? 90% specificity is good enough.
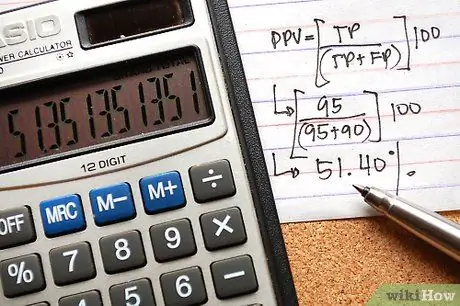
Step 8. To calculate the positive predictive value (NPP), divide TP by (TP+FP)
In the above context, the calculation is 95/(95+90) = 51.4%. A positive predictive value tells the probability of a person having the characteristic if the test result is positive. Among all those who test positive, what proportion actually have the characteristic? An NPP of 51.4% means if your test result is positive, the probability of actually suffering from the disease in question is 51.4%.
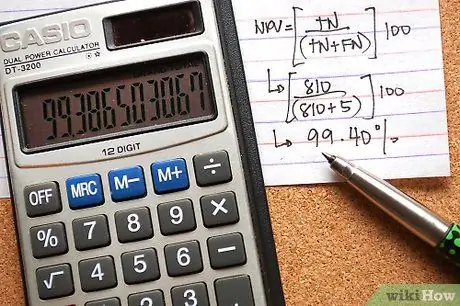
Step 9. To calculate the negative predictive value (NPN), divide TN by (TN+FN)
For the example above, the calculation is 810/(810+5)= 99.4%. A negative predictive value tells how likely a person is not to have the characteristic if the test result is negative. Among all those who test negative, what proportion actually lacks the characteristics in question? NPN 99.4% means that if a person's test result is negative, the probability of not having the disease in that person is 99.4%.
Tips
- Accuracy, or efficiency, is the percentage of test results correctly identified by the test, i.e. (true positive + true negative)/total test result = (TP+TN)/(TP+TN+FP+FN).
- A good screening test has high sensitivity, because you want to be able to get everything that has certain characteristics. Tests that have a very high sensitivity are useful for ruling out a disease or characteristic if the result is negative. ("SNOUT": SeNsitivity-rule OUT)
- Try to make a 2x2 table for more convenience.
- Understand that sensitivity and specificity are intrinsic properties of the test that no depends on the existing population, i.e. that the two values should be the same if the same test is performed on different populations.
- A good verifiability test has high specificity, because you want the test to be specific and not mislabel people who don't have the characteristic by assuming they have it. Tests that have a very high specificity are useful for enclose certain diseases or characteristics if the result is positive. ("SPIN": SPecificity-rule IN)
- The positive predictive value and the negative predictive value, on the other hand, depend on the prevalence of this characteristic in a particular population. The rarer the characteristic sought, the lower the positive predictive value and the higher the negative predictive value (because the pretest probability is low for rare characteristics). On the other hand, the more common a characteristic is, the higher the positive predictive value and the lower the negative predictive value (because the pretest probability is high for the common characteristic).
- Try to understand these concepts well.